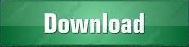
This violates the assumption of homoscedasticity. But the scatter plot shows we do not have a constant variance: As year increases, the spread of scores gets wider. We see a significant relationship such that, for every year that passes, we expect about a 0.1 decrease in rating. # Residual standard error: 1.081 on 391 degrees of freedom # lm(formula = rating ~ year, data = ratings) Ggplot(ratings, aes(x = year, y = rating)) + This leads to an obvious research question: Do I think movies are getting better-or worse-over time? I start my bringing in my data and regressing rating on year: library(tidyverse)
CONEXANT SMARTAUDIO HD WINDOWS 10 TOSHIBA 2016 KEYGEN

It also exports the year in which the film was released. Letterboxd has an amazing feature that allows you to export your data as a. I started doing this at the beginning of the pandemic, and I been watching a lot of movies throughout this time of social distancing. Letterboxd is an app that I use to log the films I’ve watched: It keeps track of when I watched a film, how I rated it (0.5 to 5.0 stars), and any text review I gave it on that watch. The use case here are my Letterboxd ratings.
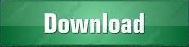